From robotic perception and manipulation to self-driving cars, machine learning typically requires large data sets that are laboriously annotated by humans. Figure Eight Inc. today announced Workflows, a feature it said “automates the creation of complex data-annotation jobs at scale.”
“Workflows makes it possible for non-technical users to create granular, plug-and-play, multi-step annotation projects, removing bottlenecks and lowering the cost of data annotation across the board,” said Figure Eight. Workflows also provides the flexibility to target different contributors for every annotation step, so that only highly skilled contributors handle the most difficult steps, it added.
“Overly complex data-annotation jobs increase the cognitive load on the global crowd tasked with labeling vast quantities of training data,” stated Wilson Pang, chief technology officer at Appen Ltd. Australia-based Appen, which develops human-annotated data sets for artificial intelligence, acquired San Francisco-based Figure Eight for $175 million last year.
“To help create high-quality machine learning data more effectively, we’ve developed technology that streamlines the annotation process,” Pang said. “Workflows easily connects multiple, more specific jobs within large annotation projects to optimize the process for quality and improve the experience for both AI experts and the annotation crowd.”
“By creating more granular annotation jobs, Workflows also delivers high-quality results faster, leading to fewer wasted resources and reduced costs when compared to large, complex annotation jobs,” he added. Machine learning-assisted data labeling (MLADL) combines human annotation with machine learning to deliver annotated data up to 20 times faster at up to a 50% lower cost, claimed Figure Eight.
Connecting the training data pipeline to human annotation
“I was a product manager at IBM Watson, and as we scaled AI service for stuff like computer vision and natural language processing, we needed a lot of annotated data — hundreds of millions of dollars worth,” recalled S. Alyssa Simpson Rochwerger, now vice president of product at Figure Eight. “I thought, ‘Hey, if I’m having this problem at IBM, I bet others are having similar problems with inefficiencies.”
“I joined Figure Eight to make the act of data annotation more efficient by applying automation, really helping the industry scale,” she told The Robot Report. “Workflows is a perfect extension of solving my own problem, linking parts of that pipeline from a data science perspective. It’s collecting data, annotating it, connecting with models, and training the models. When the models have low confidence, it’s annotating more data to retrain them.”
“There’s nothing like Workflows on the market that ties the model-training pipeline to human annotation,” Simpson Rochwerger said.
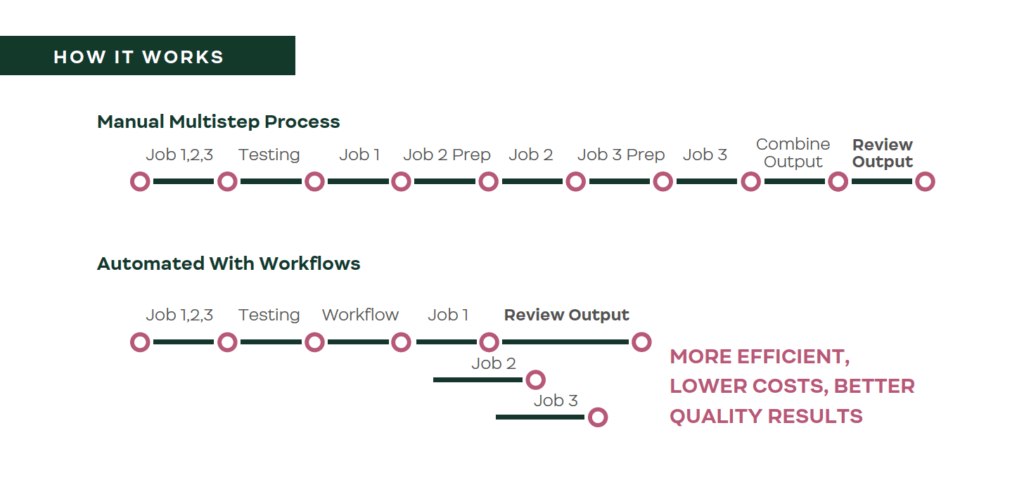
Workflows automates parts of the data process. Source: Figure Eight
Workflows starts with data-routing rules
“Workflows has routing rules for data — such as routing certain images to a second determination or to a human, based on the confidence level,” said Simpson Rochwerger. “Why that hasn’t been done before beats me. I desperately needed it when I was a practitioner.”
“There are other platforms that implement this in a narrow way or a method that’s specific to one business, such as Amazon’s ground truth. Google has it in crowd-compute models,” she said. “We decided to do it for all kinds of data — images, speech — not just simple image annotation.”
“Clients have workflows that take four screens and 40 to 50 steps,” Simpson Rochwerger said. “Without a platform like Figure Eight Workflows or Appen’s Labor Pool, they have to go to a fragmented market. There are lots of BPOs [business process outsourcers], and others are sending spreadsheets, but there are no APIs [application programming interfaces] for automation.”
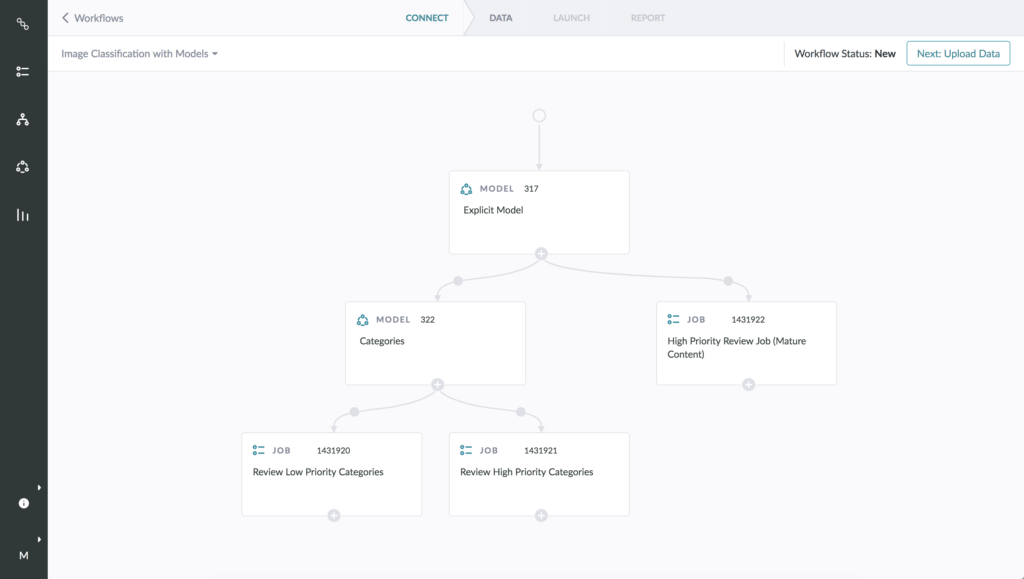
An example data-annotation process in Workflows. Source: Figure Eight
Ease of use for businesses
Workflows’ graphical user interface is designed for plug-and-play operability, allowing someone who is not a data scientist to configure operators with routing rules, said Figure Eight, which has more than a decade of experience and was formerly known as Dolores Lab and CrowdFlower.
“Often, launching a model is terrifying for a business user, who doesn’t know who it will perform in a production environment,” said Simpson Rochwerger. “The Workflow platform is a good way to link real production data to a backstop of human labor in close to real time.”
“In many cases, clients were building out custom workflows with data scripting outside a platform, so Workflows can save a lot of time,” she said. “Breaking down an individual annotation task, such as marking a tree in an image, and adding a second step of peer review or asking what type of tree it is requires specialization. You want higher quality and consistency, where the model has 90% confidence, and the rest is fed to humans and back to the model. Business users can adjust those dials.”
Use cases for Figure Eight Workflows
Artists upload more than 4,000 assets to the Society6 online community every day, and it must filter out low-resolution images, as well as inappropriate or copyrighted material. Workflows automated the process of separating items into review buckets, helping Society6 avoid legal problems and enabling its internal team to review almost 30,000 pieces in two months, up from a few thousand pieces per month.
“Society6 was an early adopter, with a platform similar to Etsy,” said Simpson Rochwerger. “If the confidence level is not higher than, say, 85%, the data is routed back to a human to annotate. It then goes back into the model, using an IBM visual recognition system, for training with the new data.”
“In the case of robots, which are interacting with something, that confidence level needs to be high,” she acknowledged. “Our robotics customers are in the back-office space, agriculture, and assistive devices in the home. Their robots need to grasp objects, do household chores, or plant seeds.”
Figure Eight has conducted more than 10 billion judgments, and its customers include Tesco, eBay, Oracle, and Bossa Nova Robotics, which is expanding its mobile robot deployments to 1,000 Walmart stores.
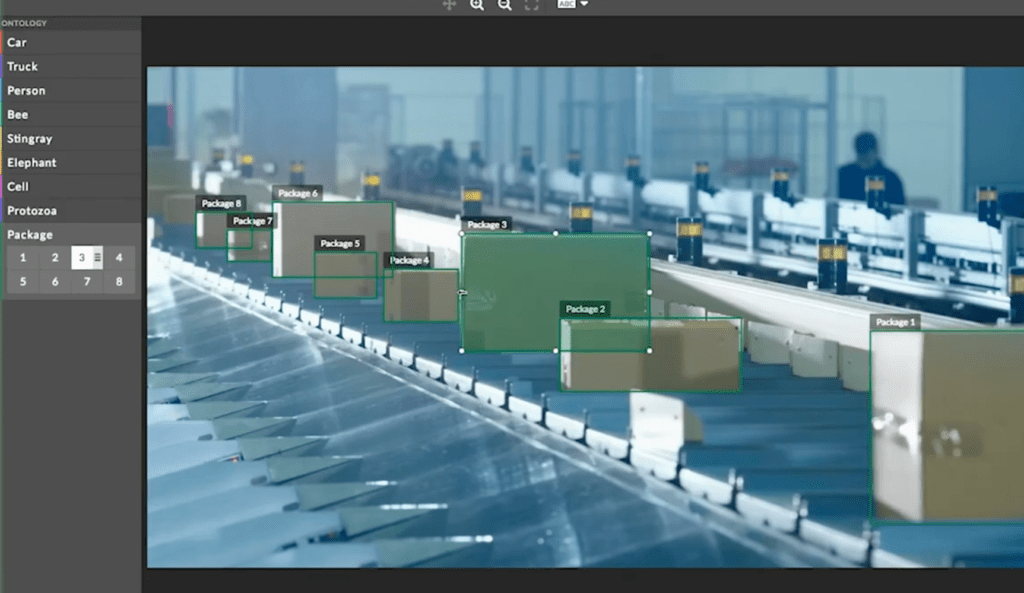
Workflows is designed to make data annotation easier, more accurate, and more affordable. Source: Figure Eight
Internationalization aids quality of annotation
“Multinationals need access to a variety of complex uses cases, multiple languages, and skills,” noted Simpson Rochwerger. “To automate the restocking of shelves, you need diversity of ingested data, as well as diversity of people labeling that data to understand what the products are. We’re rolling out worldwide, so depending on language, Workforce can route data to different pools of annotators.”
“Another way of doing it is you can have data come in and send one half to one set of people and the other half to another,” she said. “A business owner can create narrow models that look only at specific features and change thresholds and change them based on their risk tolerance.”